Concept: Randomness in Business Systems
We encounter many variables that appear to change randomly. In most situations, randomness is a measure of our ignorance, which is not intrinsic to the system. (Except in quantum mechanics, where Einstein’s famous lament ‘God does not play dice with the universe!” appears to be wrong. However, the random behavior of elementary particles near the Planck scale has little or no bearing on the dynamics of macroscopic systems, such as a company).
In a business context, when we for example say that there are ‘random’ variations in the demand for a firm’s product, what we actually mean is, that we do not know the reasons for these variations. We are only revealing the limitations of our understanding and not characterizing a feature of reality. The demand may be growing or declining, or it may even be seasonal. The firm may understand the patterns at a macro level and forecast the trend or the seasonal cycle with some account of accuracy. However, after accounting for all sources of change, the residual variation still persists which people call random, as the manager’s model of consumer behavior is imperfect. It is practically impossible to account for all local conditions and idiosyncrasies of customers. If the knowledge of consumer behavior had been perfect, then the total variation would have been structured into the model of the system.
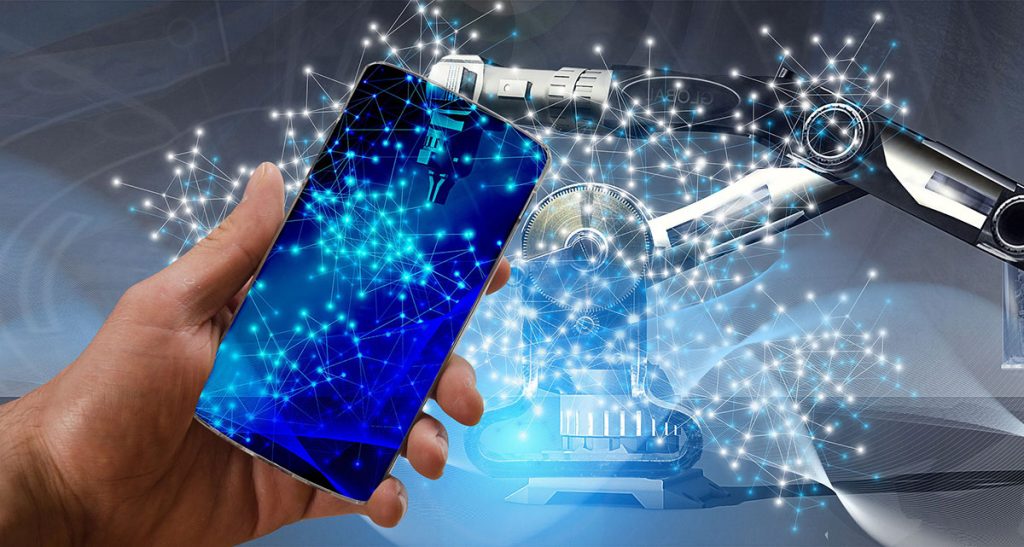
The fluctuations arise due to the deviations of the aggregate behavior of individuals from the statistical average behavior. This results in systems being bathed in a rain of random shocks with occasional downpour or floods, as we are experiencing in the current economic crisis resulting from the pandemic. Such situations are prevalent across all kinds of systems, particularly in business systems operating in unpredictable environments resulting from turbulences. The random shocks affect the performance of all core and support processes—sales & marketing, production, supply chain, product development, customer support, human resources, finance, etc.
To overcome the problem of imperfection in our knowledge, Dr. Deming, the Guru of Management and Quality, in the 1990s had introduced a new system of thought called the ‘System of Profound Knowledge’. He urged business professionals to view the world simultaneously through four lenses—Appreciation of Systems, Knowledge of Variation, Psychology, and Theory of Knowledge—the pillars of the knowledge system. The fifth pillar—Knowledge of Systemic Waste Reduction—was added to integrate the Japanese philosophy and methodology based on Lean Management, pioneered by Toyota. The five pillars systematized the approach of minimizing variation and wastes in a scientific and systematic manner, based on a statistical analysis of data and the logic of systems-based management where value creation from standpoint of customer & stakeholders, was the main purpose of business. It resulted in the integrated and structured methodology for business performance improvement in the first decade of the 21st Century, called Lean Six Sigma (LSS).
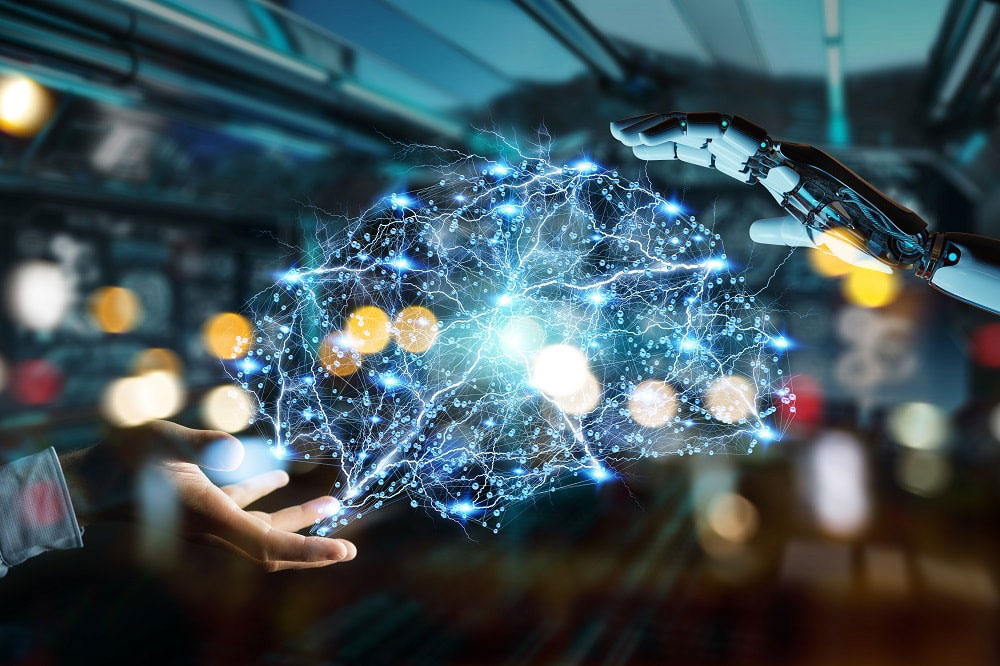
Context: Counterintuitive Behavior — Automotive versus Aerospace Manufacturing Systems
LSS made a radical impact on the competitiveness of a range of businesses, the principles practiced extensively by a wide range of companies in different sectors. It improved their process and product performance, driving up both revenue and profitability simultaneously along with the reduction of costs, as companies globalized and expanded their markets with increasing complexity in their operations as value chains became more complex. Data Science was at the heart of LSS, however, dominated by statistical tools for analysis and diagnosis, for characterizing the system and the problems. The logic of business process improvement was applied based on data analysis and diagnosis using statistical methods that revealed patterns at an aggregated & macro level. For example, the Pareto principle (80:20) of vital few versus trivial many was used to prioritize actions for improvement based on an established pattern or rule that systems usually follow provided by statistical science.
The business logic for revenue maximization based on Pareto worked well for automotive applications to eliminate the systemic wastes such as time lost during the changeover of the production line setup as it is switched from one product to another. The pattern of vital few factors that affect systemic wastes or variations follows the Pareto rule, where manufactured parts are of high volume, high production frequency—highly repetitive and of low variety. The 20% vital few parts that go into automobile manufacturing, accounted for 80% of the revenue. The set-up time reduction of the production lines for this vital few meant higher flexibility of their production lines and capacity to cater to a greater variety of products, called the Single Minute Exchange of Dye (SMED), by Toyota. The practice of SMED helped Toyota to achieve breakeven at a fraction (25%) volumes compared to GM and Ford. Hence LSS method using Pareto and other techniques can help us to see the systemic wastes and eliminate them leading to value maximization from the business processes.
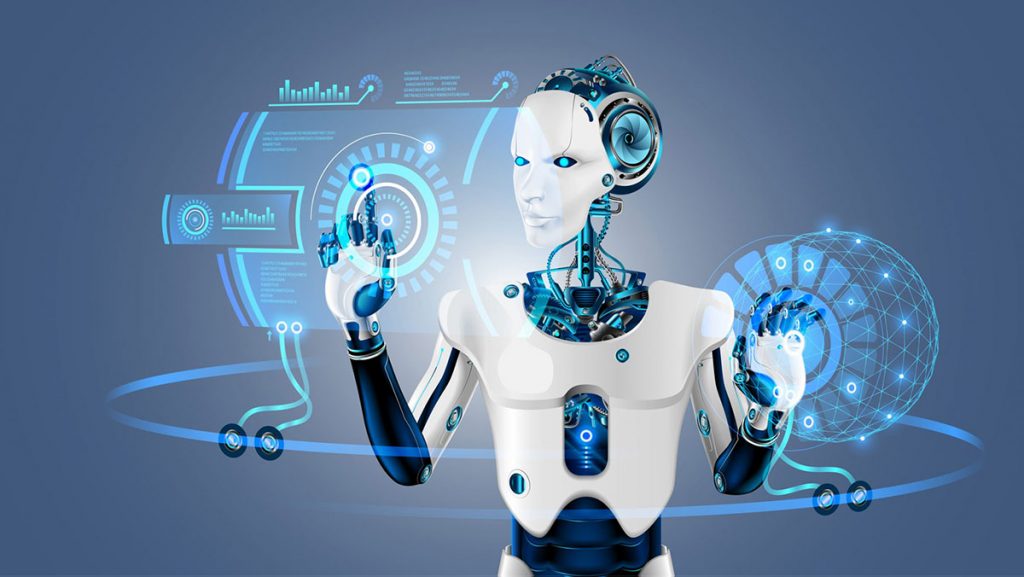
However, the same logic when applied to Aerospace for eliminating systemic variation or wastes proves to be counterproductive due to a fundamental contextual difference compared to the systems in automotive. This sector manufactures low volume, low production frequency, or non-repeatable and high variety parts for their various systems and subsystems. For example, the landing gear of an aircraft has about 1000 parts. The business logic of applying the Pareto principle of vital few for revenue maximization meant a focus on about 180 parts that contributed to 80% revenue. The balance of 820 parts contributed only 20% of revenue. However, the set-up time of the assembly line resulted in inventory piling up and cash flow issues.
To figure out this deviation from the accepted normal behavior, AI-based on Deep Neural Network (DNN) technology was used and it surprised all by revealing an underlying pattern that was totally counterintuitive to the prevailing thinking. It was revealed that the 820 parts, though making only 20% contribution to revenue, were actually taking up about 73% of the total set-up time, therefore in effect reducing the production capacity of the plant. Hence, only AI-based systems can see the deep underlying micro patterns and structures that LSS cannot see and decipher. Thus, the attention had to be given to this trivial many, under such conditions where statistical models do not reveal the true picture! The counterintuitive behavior of the complex Aerospace production system could be revealed only when the setup time patterns of every part at a micro-level were analyzed and common patterns were revealed to include all parts. This resulted in a completely different optimization strategy taking into account the different contexts that resulted in the complexity of the aerospace production process which is different from the automotive industry.
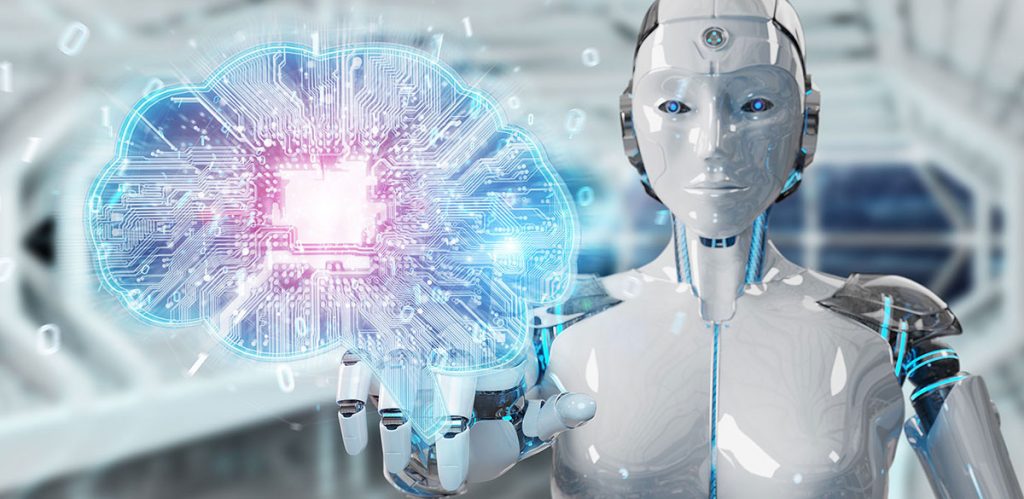
Conclusion: Benefit of AI and Future Practice
AI-DNN unleashes the power for us to understand the deeper underlying interrelationships between the factors affecting the patterns of behaviors in systems, which cannot be revealed using conventional logic, analytical or statistical methods, and tools. The advantage of AI is that it can unravel the specific context under which the system’s behavior is influenced in almost real-time, making it resilient. As the scale of complexity increases, the power of AI-DNN also multiplies, now enabled by technologies such as Cloud Computing and IoT, to leverage data at a global level. The future is moving towards greater personalization of strategies through deeper contextualization and customization of products and services offered to customers. The AI methods can see what conventional methods can’t and hence moves the capabilities one step ahead, by delivering real-time action from the insights.
The neural network-based AI structures can dive deep to unfold the hidden patterns of behaviors of Man and Machines and the systems involving them in a dynamic fashion. Therefore, this dynamic capability that AI adds to LSS, multiplies the diagnostic power, hence the effectiveness of process improvement methodologies such as LSS enhances by focusing the methodology quickly on to the right problem. The time is also not too far when Neural Network based AI coupled with the power of Cloud Computing and IoT would be able to predict the demand and consumer behavior so accurately, that the process of ‘Shipping before Selling’ would become a defensible business model which no competitor can counter!
***